Artrya Salix for Physicians
Screen for atherosclerosis and plaque features in minutes
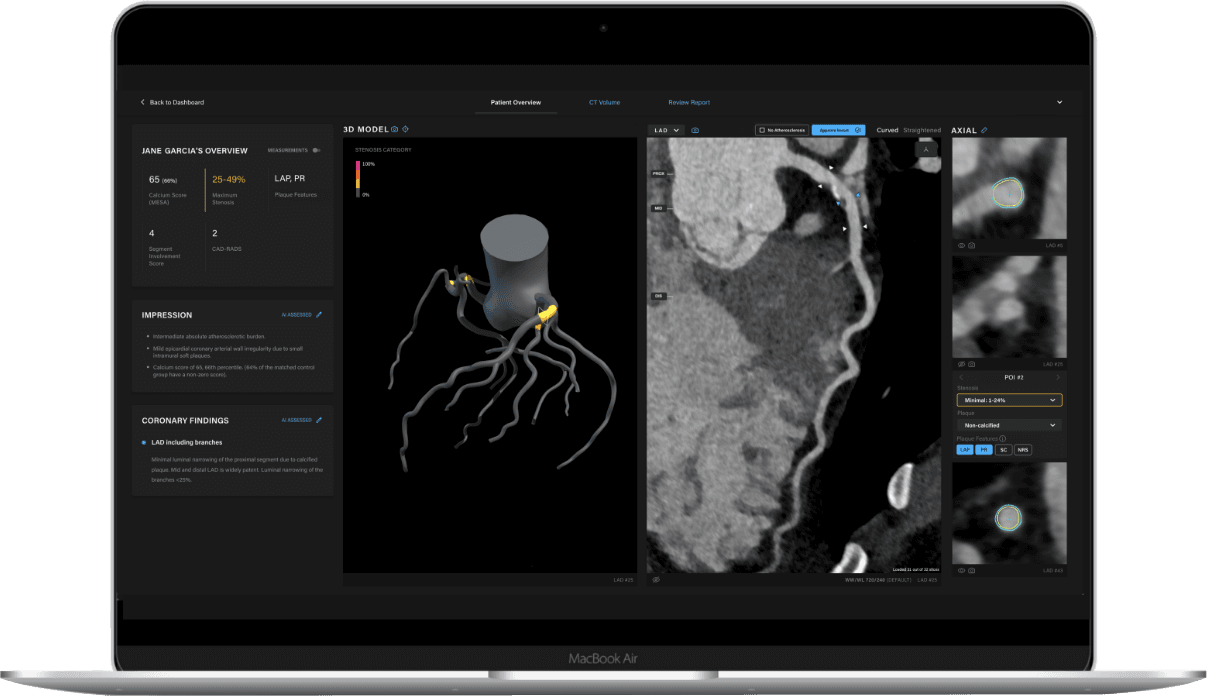
With the power of AI, it is possible to accelerate the triage of chest pain patients to prevent unnecessary hospitalization and prolonged diagnostic testing of patients with non-critical disorders, while rapidly identifying patients at risk of heart attack.
Artrya Salix is a CCTA image analysis solution that empowers physicians with AI to identify and analyze the extent and type of arterial plaque. This fully integrated solution uses no external reading teams to validate cardiac scan data, allowing physicians direct control of their AI-supported patient scans within minutes. This technology will revolutionize the speed and accuracy of coronary artery disease diagnosis.
Precision diagnosis with Artrya
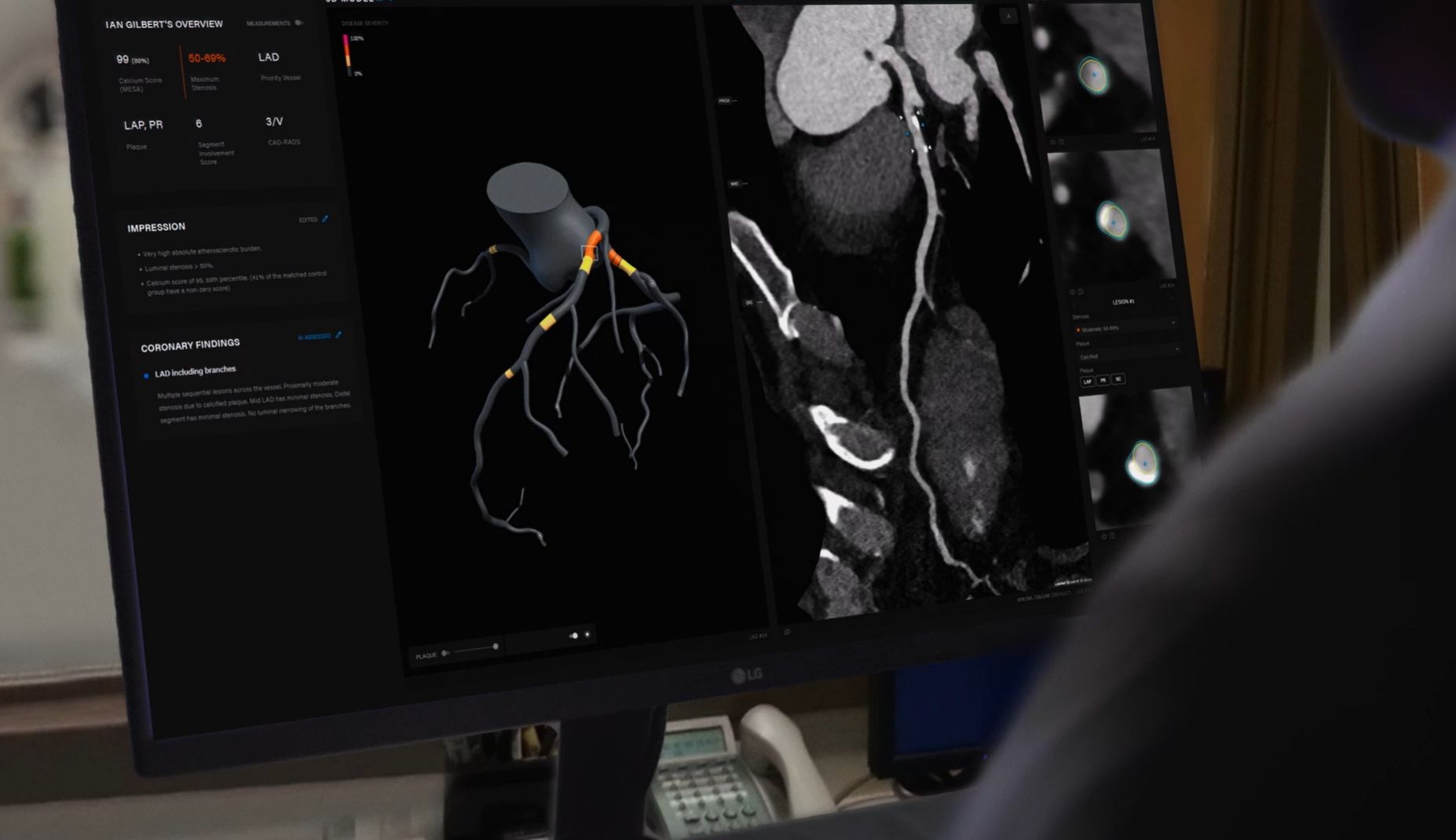
Let’s change the statistics
Artrya provides a tool that streamlines the effective use of CCTA as a first-line diagnostic test for CAD. A tool that standardizes data and reporting based on SCCT guidelines to facilitate collaborative care between interpreting and referring physicians, providing better treatment planning, intervention analysis and referral of patients to the appropriate level of care.
Shining a light on the value of plaque type
Dr. Yasmine Ali, Cardiologist, TennesseeFor much of the past century, ischemic heart disease has been the leading cause of death worldwide. The rupture of atherosclerotic plaques and subsequent thrombi formation are the etiologies of the majority of acute coronary syndromes. 4 Accurate detection of these high-risk plaques, therefore, has the potential to prevent global morbidity and mortality when coupled with effective preventive therapies.5
High-risk plaques have been recognized to have distinct morphologies, such as thin-cap fibroatheromas with their lipid-rich necrotic cores, plaque erosions, intraplaque hemorrhage, microcalcifications, and positive remodeling.5 Identification of these plaques, however, has traditionally been possible only through invasive imaging modalities such as intravascular ultrasound (IVUS) and intravascular optical coherence tomography (OCT).6
Non-invasive imaging techniques such as CCTA have been evolving for the detection of high-risk plaques7 and presenting the known morphological features of high-risk plaques as they appear on CCTA can lead to more robust detection and diagnostic reliability.8
Once high-risk plaque is identified, preventive therapies can be tailored to the patient individually, allowing for a higher level of precision in treatment and care. Treatment with proven lipid-lowering and anti-inflammatory therapies, for instance, along with heart-healthy lifestyle changes, can stabilize plaques and reduce the risk of future cardiovascular events.9
Artrya Salix Image Analysis: advancing diagnosis with machine learning
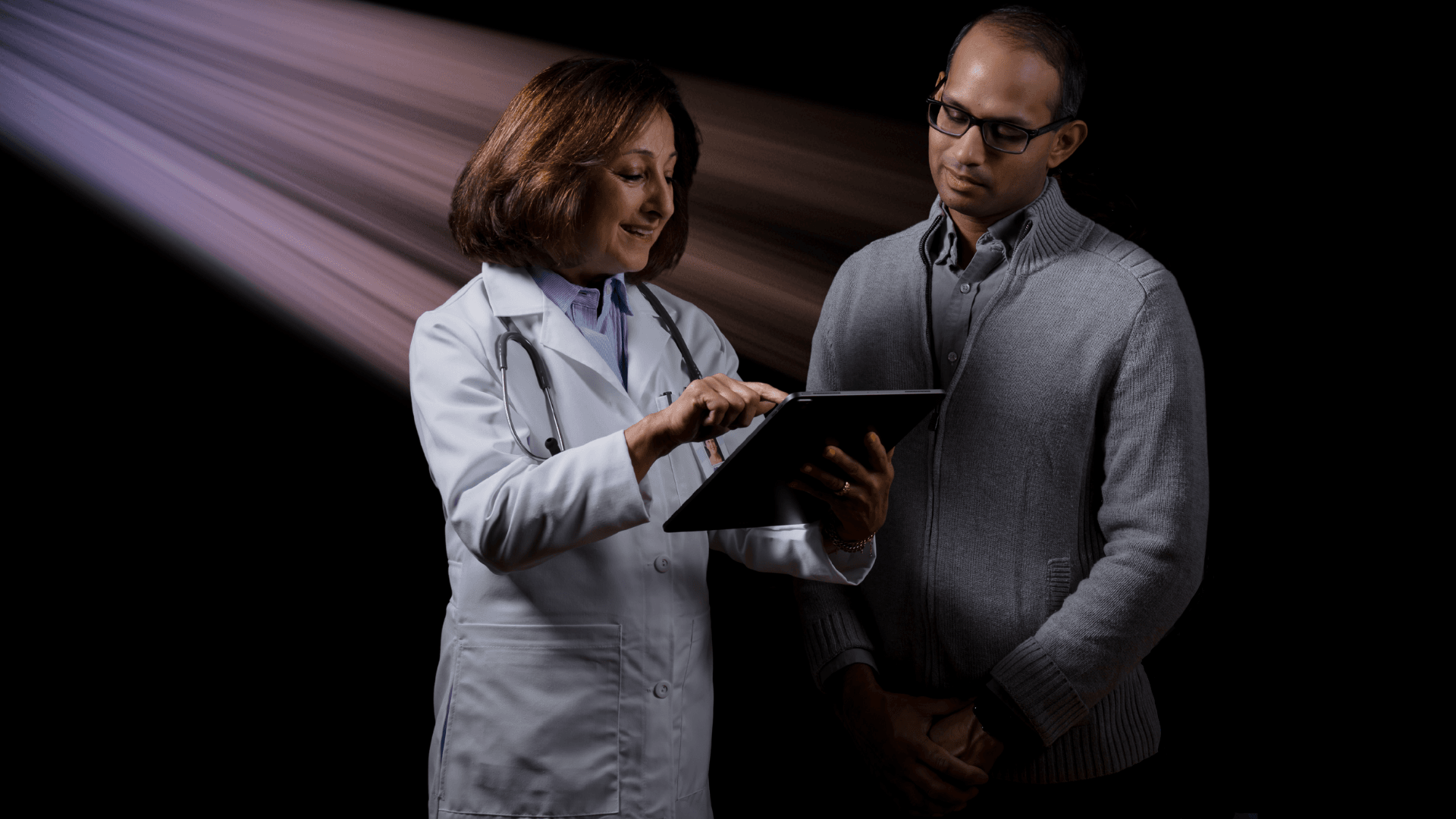
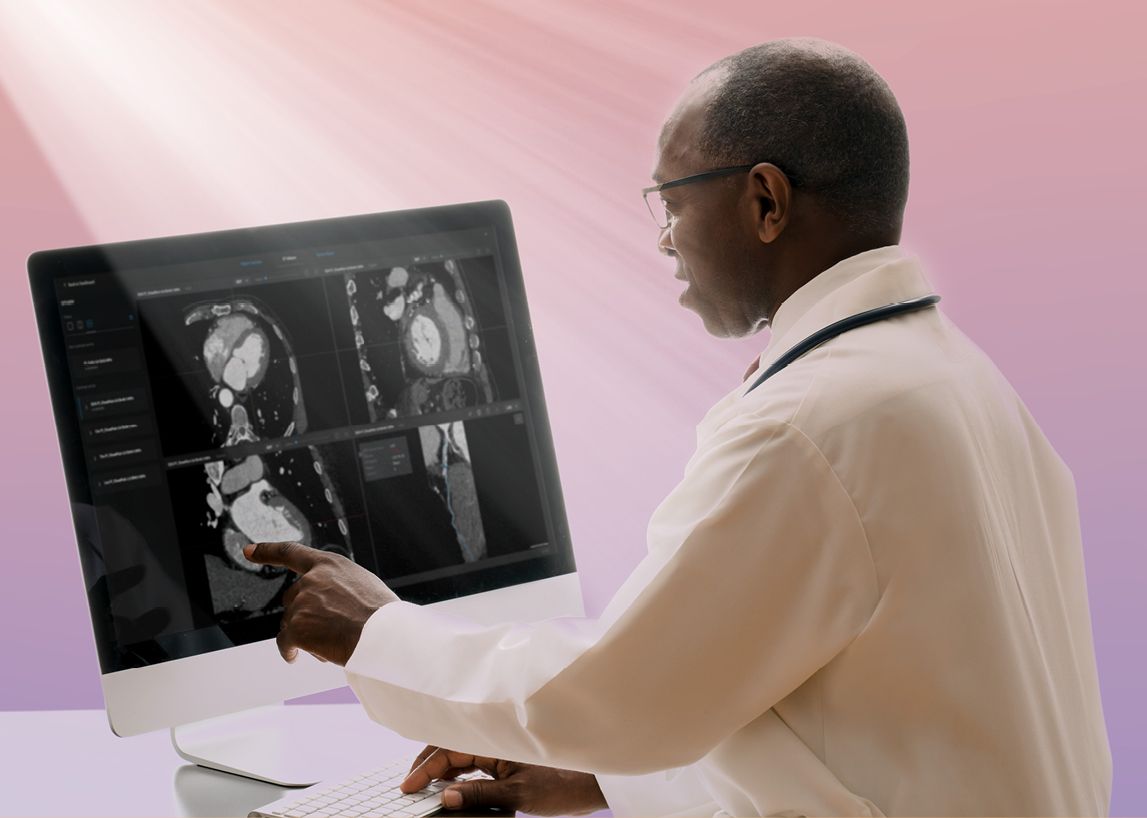
The key to scan analysis is the segmentation of the inner and outer walls of the coronary arteries. In this process, we identify and track the centerlines of the arteries.
Two convolutional neural network (CNN) based deep learning models are used to extract centerlines: a coronary artery seed detection algorithm and a centerline tracking CNN. Of importance, tracked arteries terminate at the detected aorta to eliminate false positives. Following centerline extraction, vessel wall segmentation projects the original CT scan into straightened multiplanar reprojection (sMPR) relative to each coronary artery.
To maximize the analysis of coronary artery disease indicators, stenosis is estimated by assessing the relative surface area of lumen cross-sections relative to a healthy reference, determined by identifying disease-free areas of the vessel proximal and distal to the lesion. Plaque features such as low attenuation plaque (LAP), positive remodeling and spotty calcification are detected by analyzing the spatial and radiometric characteristics in the region between the inner/lumen and outer walls of extracted coronary arteries. Plaque volume measurements are calculated by identifying voxels that are within the target Hounsfield Unit (HU) ranges and are spatially located between the inner/lumen and outer wall of the coronary arteries.
The algorithm has been built from access to thousands of scans from world-renowned medical institutes in the US, Australia and Canada for impressive accuracy.
Physician-led, AI-based CCTA analysis coupled with an intuitive and easy-to-use clinical reporting platform can save physicians valuable time that can be used to educate, engage and collaborate with patients instead.
Rapid assessment. Accurate diagnosis.
References:
- Williams, 2020
- Villines TC and Williams MC. The Fallacy of the Power of Zero. J Am Coll Cardiol Img. Study link. Published May 11, 2022.
- Nair R, et. al. Characteristics and Outcomes of Early Recurrent Myocardial Infarction After Acute Myocardial Infarction. Journal of the American Heart Association. 2021;10(16). Study link.
- Benzton J F, (2014) Mechanisms of Plaque Formation and Rupture. Circulation Research Compendium on Acute Coronary Syndromes. DOI: 10.1161/CIRCRESAHA.114.302721
- Hafiane A. Vulnerable Plaque, Characteristics, Detection, and Potential Therapies. J Cardiovasc Dev Dis. 2019;6(3):26. Published 2019 Jul 27. doi:10.3390/jcdd6030026
- Lv, R., Maehara, A., Matsumura, M. et al. Using optical coherence tomography and intravascular ultrasound imaging to quantify coronary plaque cap thickness and vulnerability: a pilot study. BioMed Eng OnLine. 2020;19(90). Study link
- Canu M, Broisat A, Riou L, et al. Non-invasive Multimodality Imaging of Coronary Vulnerable Patient. Front Cardiovasc Med. 2022;9:836473. Published 2022 Feb 24. doi:10.3389/fcvm.2022.836473
- Dwivedi A, Al'Aref SJ, Lin FY, Min JK. Evaluation of Atherosclerotic Plaque in Non-invasive Coronary Imaging. Korean Circ J. 2018;48(2):124-133. Study link
- Thomas, IC, Forbang, NI, Criqui, MH. The evolving view of coronary artery calcium and cardiovascular disease risk. Clin Cardiol. 2018; 41:144– 150. Study link